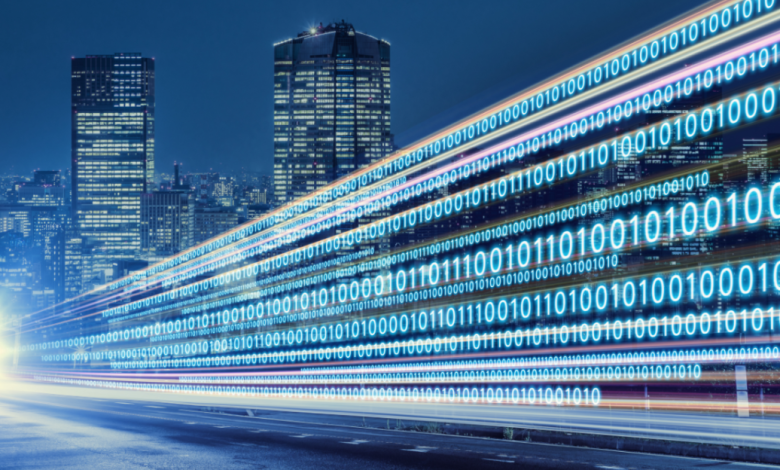
Self-service analytics provides business users with dynamic reports and the ability to evaluate data using sophisticated built-in capabilities. This type of user empowerment decreases the need for IT personnel. Furthermore, self-service business intelligence (BI) capacity may provide users with external data access as well as built-in functionality for producing finished reports quickly. Users do not need to know anything about the underlying programming layer of the applications to use the full range of analytics or BI functionalities. (data science course Malaysia)
Powerful Analytics Use Cases provides a compelling argument for “self-service analytics platforms,” which, according to the author, have the potential to be advanced analytics tools on the desktop of the average user.
Market for Self-Service Analytics (data science course Malaysia)
Through 2022, the self-service BI market in North America is expected to be the most dominant. This industry has been propelled by the increasing adoption of big data and the rise of data-driven enterprises, but security breaches, a lack of clean data, and high investment costs are all major downsides of the technology.
When it comes to choosing the right solutions for their businesses, business owners face numerous challenges, such as locating the right tools to monitor all aspects of their operations, locating the right functions to measure KPIs, and understanding the right methods to analyse performance outcomes. Understanding how to collect data from many operational processes and organise it on a uniform platform for future analysis is another key problem.
How much they allowed human data entry or coding, what platform integration capabilities are accessible, are routine solutions readily available, and is the software scalable are some of the frequent worries of a corporate buyer.
Increasing the level of trust between IT and business users (data science course Malaysia)
Although the razzle-dazzle of neat tools, bright dashboards, or multimedia reporting capabilities may entice CEOs or business leaders, it is wise to consult seasoned IT staff in the purchasing decision. A excellent strategy is to give in-house technical experts a “wish list” of technology requirements, and they may then scour the market for solutions that satisfy those requirements.
“By 2019, self-service analytics and BI users will provide more analysis than Data Scientists,” according to a Gartner news release.
Use Cases for Self-Service Analytics
Automated algorithms, which have the ability to learn from historical data and apply that learning to pattern recognition for future predictions, were once considered “advanced analytics,” but have now crossed over into self-service analytics territory. A self-service analytics platform’s success hinges on both the model generation and model deployment phases. In most circumstances, business users prefer a simple “predictive model” or a few of them to make decisions that are superior to their current technological capabilities.
Business users will increasingly want to do more than just share data and view automated dashboards after the initial changeover. Users will want to learn how to examine reports or outcomes in the middle of a project and turn them into actionable decisions quickly. As a result, ease of use, navigation, auto-update functionality, and easy interface with other programmes are among the top needs of popular self-service analytics platforms.
Healthcare Self-Service Analytics
Information Builders’ Chief Innovation Officer, Rado Kotorov, believes:
“[O]rganizations have the chance to delve deeper to unearth crucial indicators and metrics, study data and expose its potential, engage with real-time data, automate the scheduling and delivery of critical information, and leverage the resultant insights to generate improved business outcomes.” Data scientists and other elite groups no longer have exclusive access to analytical findings.”
In a survey that examined quality patient treatment at an affordable cost, the United States ranked 50th out of 55 industrialised countries in “effectiveness of healthcare delivery.” The analysts discovered that constraints such as rigid data frameworks, latency, system complexity, and understaffing might present severe impediments to effective healthcare delivery while working with healthcare data troves. The most major stumbling block is frequently seen in electronic health records (EHR), which can result in decision bottlenecks and poor outcomes.
More..
All of these problems are significantly decreasing with self-service healthcare analytics, allowing healthcare providers to immediately access, analyse, and process patient data in a reasonably short amount of time without having any Data Science experience. They automated “Data access, preparation, analysis, and reporting” in most self-service analytics packages meant for the healthcare business. Self-propelled, insight-driven healthcare systems can help with accurate diagnosis, timely treatment decisions, better treatment outcomes, and value-added healthcare delivery because of their flexibility.
Another area where industry insiders still have doubts about the reliability of stored data in the healthcare industry is data governance. According to The Impact of Data Governance on Self-Service Analytics, the phase of “data preparation” is largely or entirely automated in self-service analytics, indicating “that this technology may potentially serve as a driver for higher Data Quality and Data Governance, rather than an inhibitor.” The freedom from dependency on IT professionals, user empowerment, and precise decision-making are the most evident benefits of a self-service healthcare analytics platform.
Marketing Self-Service Analytics
Any sales team can use the Looker Sales Analytics system to filter “leads or deals” with “actionable data.” Enterprise customers can collect and view all data through a unified Data Management platform using Looker’s Sales Analytics platform. This unique application is giving “custom data experiences,” according to the article Building on the Platform: Custom Applications for Sales & Marketing.
Manufacturing Self-Service Analytics
Business operators in the manufacturing industry rely on “Six Sigma,” (also known as “Define Measure Analyze Improve Control Cycle [DMAIC]”), which serves as the key quality standard. We now may used his quality tool in conjunction with an enterprise-grade industrial analytics system to transmit findings from one unit or plant to other units throughout the company, formalising and duplicating operational excellence.
To achieve ongoing quality improvements, we integrated Six Sigma with data-driven applications. The industrial analytics system, when correctly planned and executed, can assist any manufacturing plant in achieving cost efficiency, a low carbon footprint, and increased safety.
A Six Sigma project in a lean manufacturing context may last three to six months, during which time management must test and design performance measures in an iterative manner. Science Direct article describes implementing a self-service business analytics system to support lean manufacturing.
Source: data science course malaysia , data science in malaysia